Analysis of Housing Prices Using Our GenAI Powered Data Analysis Assistant
More than ever before, data analysis plays a pivotal role in making business decisions, but it can often be time-consuming and complex. But by leveraging Generative AI powered applications, such as our Data Analysis Assistant, uncovering actionable insights through data analysis can be made faster, easier, and more accessible to those without technical backgrounds.
The dataset analyzed below contains population density per square mile and average housing values by county throughout the United States from 2015-2024. By utilizing our Generative AI powered Data Analysis Assistant, a process that would otherwise be time-consuming – such as going through counties on a case-by-case basis or even programmatically – is expedited immensely, providing comprehensive insights at the click of a button.
All of the graphs and insights contained in this blog post were created and uncovered using Fulcrum’s Generative AI powered Data Analysis Assistant.
Regression Using AI
To illustrate the power of AI in data analysis, let’s consider a recent regression analysis of the dataset described above. The analysis involved exploring the relationship between population density, housing values, and the COVID-19 pandemic. In order to accomplish this, we simply uploaded the dataset to the application and asked “What is the correlation between housing values and population density? ” The application then output an interactive scatter plot with a regression line fit to the points (included below) as well as an explanation of the insights found.
The explanation provided by the application was used to uncover the following information:
Before the pandemic, high-density areas were synonymous with higher housing values. Urban centers, with their bustling economies and vibrant cultural scenes, attracted individuals willing to pay a premium for proximity to work, amenities, and social opportunities. The data supports this, showing that areas with a population density of 2,461.13 had housing values around $608,367.06. This trend continued during the pandemic, as many people initially sought the convenience and infrastructure that cities offer.
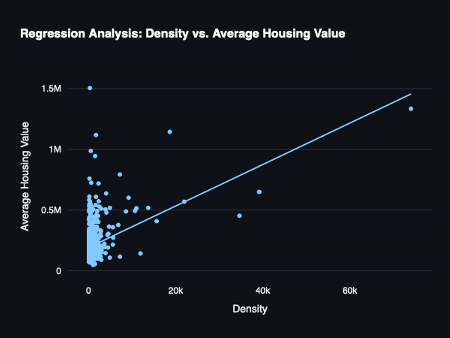
Conversely, lower-density areas traditionally had lower housing values, with a population density of 301.17 corresponding to a housing value of about $140,718.71. During the pandemic, these areas saw a surge in demand as people prioritized space and the ability to work from home. The desire for larger homes, yards, and a quieter lifestyle drove many to suburban and rural areas, leading to an increase in housing prices in these regions.
However, as remote work became more prevalent, some urban dwellers began to question the necessity of living in densely populated areas. This shift led to a temporary softening of prices in some high-density markets, as people sought more space and affordability in less crowded locales.
In addition, there is also significant variation in housing values across different densities. While there is a general trend of higher densities correlating with higher values, exceptions exist, and the trend is not very strong overall, indicating that there are more factors than just population density. This complexity underscores the need for a multifaceted approach to data analysis, which AI can facilitate by identifying patterns and anomalies that may be time-consuming if done through traditional methods.
Generally, it is more difficult to account for less tangible factors in data analysis, such as natural beauty or niceness of neighborhood. Using the assistant, however, we can see which counties are clear outliers and find out what factors they have in common. By prompting the assistant to find which counties deviate from the overall trend the most, we can generate a list that shares certain characteristics that may not be as easily quantifiable as population density.
AI-powered Case Studies
Now, let’s take a look at the counties that experienced the most significant property price increases using the Data Analysis Assistant by asking the application “Which counties deviate from the overall trend in this time period the most?”
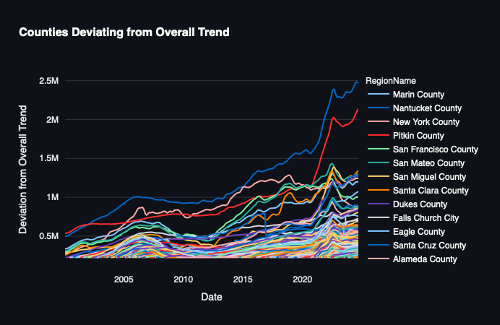
Here are the top 10 counties:
- Pitkin County: +$1.06 million
- Teton County: +$1.04 million
- Nantucket County: +$959,968
- Dukes County: +$621,796
- San Miguel County: +$621,604
- Eagle County: +$602,857
- Routt County: +$501,439
- Summit County: +$498,971
- Santa Clara County: +$463,615
- Monroe County: +$413,238
One of the most significant shifts during the pandemic was the rapid adoption of remote work. As companies realized that productivity could be maintained outside traditional office settings, employees gained the flexibility to live farther from their workplaces. This newfound freedom led many to seek out more desirable living environments, often in scenic or less densely populated areas.
Pitkin County, CO and Teton County, WY, for example, are renowned for their natural beauty and outdoor recreational opportunities. The ability to work remotely made these locations highly attractive, driving up demand and, consequently, property prices.
The pandemic also prompted a reevaluation of lifestyle priorities. Urban dwellers, confined to small apartments during lockdowns, began to crave more space and a closer connection to nature. Counties like Nantucket, MA, and Dukes County, MA (home to Martha’s Vineyard), offered the perfect escape with their picturesque landscapes and tranquil settings.
High-net-worth individuals played a significant role in driving up property prices in certain counties. Monroe County, FL, known for the Florida Keys, became a hotspot for luxury real estate as affluent buyers sought exclusive, private retreats.
Similarly, San Miguel County, CO, and Eagle County, CO, known for their ski resorts and outdoor activities, saw increased interest from those looking to balance work with a healthier, more active lifestyle.
As primary urban markets became less attractive due to high population density and the associated health risks, secondary markets gained prominence. Routt County, CO, and Summit County, CO, both known for their outdoor amenities and lower population densities, became prime targets for those seeking a safer, more relaxed environment.
Individual Comparisons
We also wanted to compare specific counties, so we asked the application to “Compare the trend between New York and Pitkin County between 2018 and 2024.”
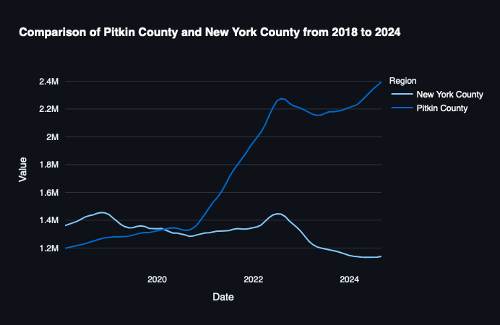
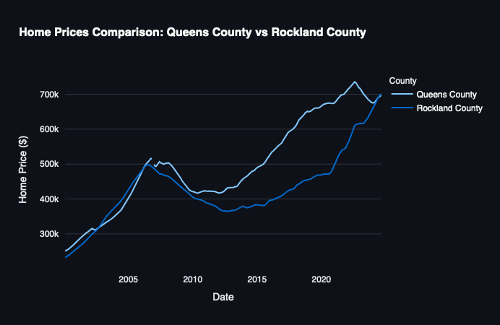
Plots created by Fulcrum’s Generative AI powered Data Analysis Assistant
As the results show, a county such as New York, encompassing Manhattan, saw a decrease in housing values during the COVID-19 pandemic due to several interrelated factors. The pandemic intensified the desire for more spacious living environments, prompting many residents to leave densely populated urban areas in favor of suburban or rural settings, thus reducing demand for city housing. Economic uncertainty, marked by job losses and business closures, further strained the real estate market, as financial instability deterred potential buyers. Additionally, the city’s dynamic housing market, characterized by high turnover, experienced an increase in available units, which, when coupled with decreased demand, led to a drop in prices. Lastly, the shift in lifestyle preferences towards remote work and more spacious living arrangements outside urban centers further contributed to the downturn in housing values in New York County.
Similarly, Queens County experienced a slight stagnation in housing value following the COVID-19 pandemic, whereas Rockland County, located in the Hudson River Valley further upstate from the city, did not. The population density for Rockland County is about 1942.41 people per square mile, while Queens is over ten times that at 21971.82 people per square mile. Housing prices for Rockland County tended to be less than Queens County before the COVID-19 pandemic but increased above it afterwards.
AI-powered data assistants are transforming the landscape of data analysis, making it more accessible, efficient, and insightful for businesses. By harnessing the power of AI via our Data Analysis Assistant application, we were able to perform an analysis and write this blog post in a fraction of the time it would have taken us to do so using more conventional methods. Additionally, performing and reviewing this analysis required a minimal time commitment from our technical data science staff allowing them to stay fully engaged in their impactful client-facing projects.
Whether you’re in real estate, finance, or any other industry, embracing AI in data analysis is a strategic move that can lead to smarter decisions and better outcomes. If you want to explore how this application or other Generative AI solutions can enhance your business, contact us today. For more insights into the world of Generative AI, follow our blog or sign up for our mailing list.