Artificial intelligence, or “AI”, is a buzzword that has been around for decades… but what does itreallymean? For most people, it can be hard to differentiate between the futuristic sounding AI and its less glamorous sounding counterpart, machine learning, sometimes called “ML”.
Even Fulcrum’s Data Scientists agree, that while the average person interacts with some level of AI or machine learning on a daily basis, it can be hard to separate the practical applications of the two. Adriano Yoshino, a Senior Data Scientist at Fulcrum Analytics, says, “I hear it all the time in conversations, people confusing the terms or using the terms AI or Machine Learning without knowing much about it. In my opinion, AI is a very vague term, everyone is using it to advertise capabilities, and the meaning is being banalized.”
Below, we tease out therealdifference between the two, as well as their intended and possible future uses.
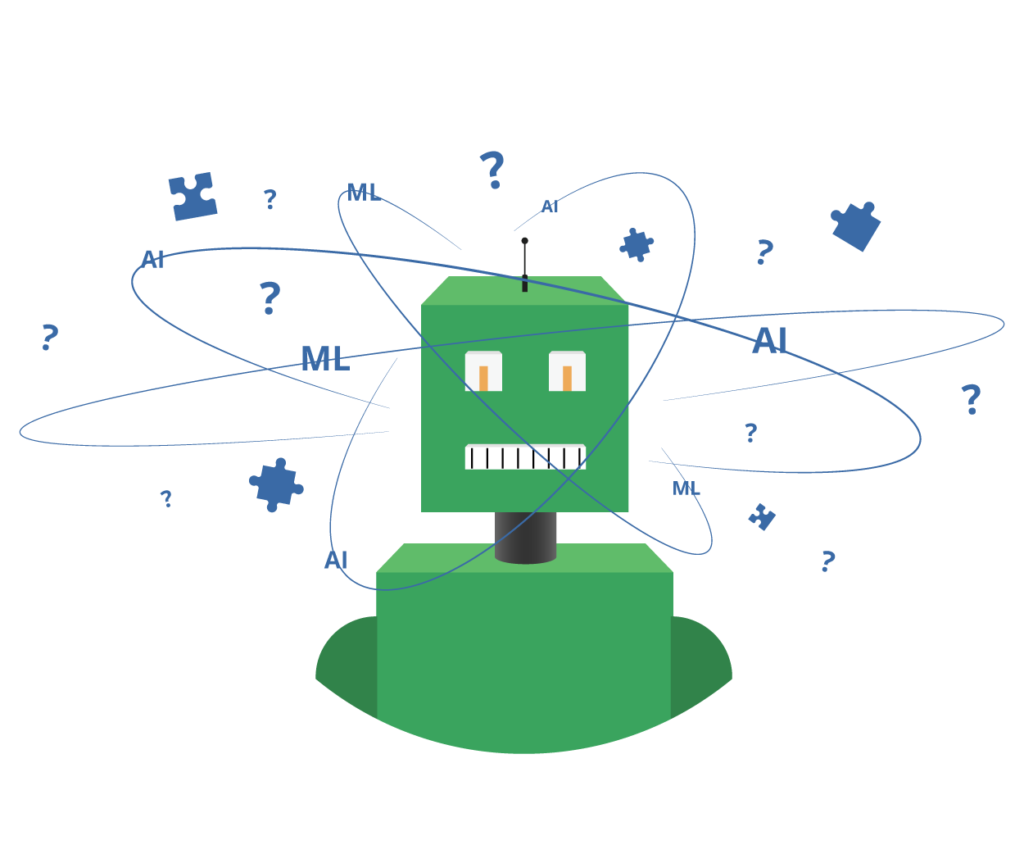
What is the basic difference?
Machine learning and AI are buzzwords that are being thrown around a lot these days: in conference names, product offerings, job descriptions, course titles, and almost everywhere else you look. The problem is, if you ask 100 different people what each term means you’ll get about 100 different answers.
Most published definitions tend to define machine learning as either an application or a subset of AI, so by the technical definitions, if you are working with machine learning, you are working with artificial intelligence.
What is machine learning and how is it used?
Machine learning is a coded process based on statistical methods which are chosen by the programmer depending on what the machine will intake. Machine learning “learns” to make predictions based on a feedback loop of predetermined correct and incorrect outcomes. The “machine” is then able to modify its future predictions based on the ingested knowledge. Examples of machine learning include:
- Spam filters, which learn from users confirming or reversing the filtered messages
- Individually curated music playlists, which learn from user likes/thumbs ups/skips
- CAPTCHAs, which learn from users ultimately proving themselves as human
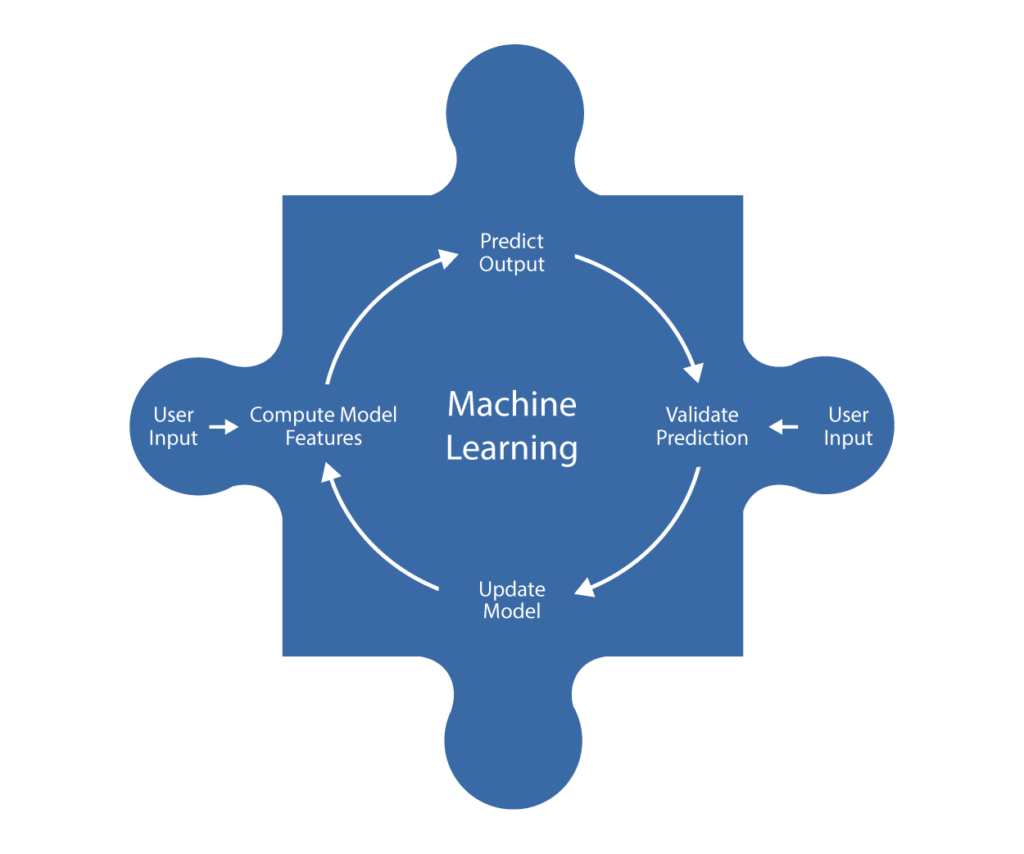
What is AI and how is it used?
About 60 years ago when AI was first emerging as a concept, the idea was to build machines that could independently think for themselves and have the intellectual complexities of a human. Today, there are two types of AI: narrow and generalized.
Narrow AI encompasses the systems that are designed to do intelligent, human-level work. Real-time traffic mapping is a great example, as the AI is using what it has been specifically programmed to do in order to alert users of relevant delays and accidents, and suggest alternate routes with less travel time. Other examples of narrow AI include:
- Artificial web monitors that detect anomalies in web traffic patterns for security purposes
- Automated online support/Chatbots that can respond to limited topics autonomously
- Robo-readers such as plagiarism scanners that detect similar patterns in written words
While narrow AI has long been integrated into our everyday lives, generalized AI is far more controversial, as it is an umbrella term for the overall goal of machines to independently judge, plan, and acquire new skills.
Generalized AI is heavily scrutinized because, in theory, machines might develop biases and intent that exceeds their initial programming and humans may lose control. So while narrow AI rests on a system of machine learning, generalized AI is as close to human as possible (which unfortunately may include the negative aspects of humans) — essentially, what we are used to seeing in popular media such as books and sci-fi movies.
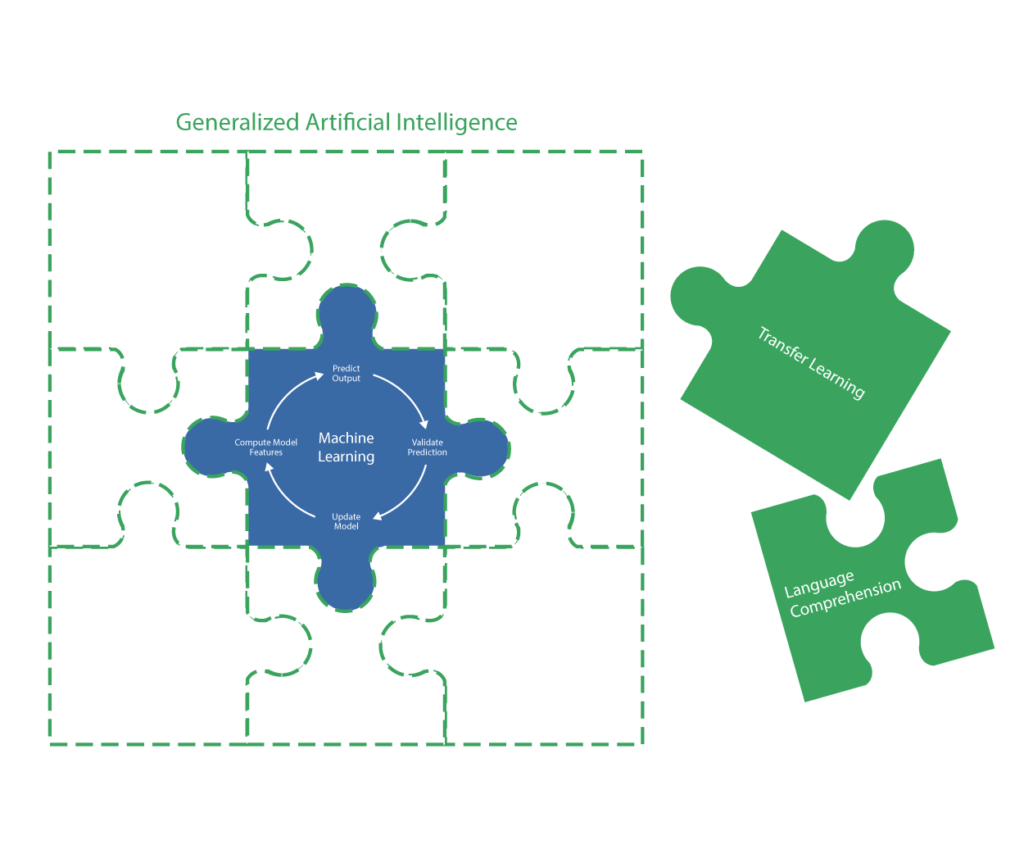
Where does machine learning end and AI begin?
It can be difficult to separate the two as they’re both quite integrated and all of AI uses machine learning for its tasks. AI attempts to simulate a specific part of human intelligence (the ability to recognize faces and objects, translate sentences from one language to another, etc.) and it does so with the use of machine learning. Think of machine learning as the foundation upon which AI is built, and generalized AI as the threshold at which computers become autonomous.
From our perspective, whether a data-driven system is termed as machine learning or AI doesn’t make much of a difference in the end. Call it statistics, analytics, data science, machine learning, artificial intelligence, or anything you like – we are in the business of making efficient use of data to solve complex business problems. If you have data processes that you think would benefit from a machine learning upgrade, please contact us and we will help you explore the possibilities.